Have you ever noticed how the same patterns and cycles repeat themselves in life? From financial markets, economic cycles, and sports performance to nature’s phenomena – cyclical analysis is an effective way of studying seemingly random data sets and uncovering trends or repeating patterns.
By understanding the mechanism behind cyclical analysis, its application in various fields, advantages & limitations, best practices for implementation, and case studies, this article will provide you with a comprehensive overview of this powerful analytical tool.
Cyclical analysis is a powerful tool for predicting future occurrences and optimizing strategies, enabling businesses and organizations to allocate resources more effectively, plan for seasonal changes, and make better-informed decisions.
Cycle Quest offers personalized cyclical analysis services tailored to businesses, investors, and curious learners’ needs. With the right domain knowledge, precise data resolution, and suitable conditions, cyclical patterns can be detected in datasets to improve decision-making.
Contact Cycle Quest today to start uncovering hidden cycles within your dataset and gain a competitive edge!
Table of Contents
What Is Cyclic Analysis?
Cyclical analysis is a data analysis technique that helps to uncover patterns and dynamics in datasets by identifying cycles of periodic fluctuations. It can be combined with trend analyses for better comprehension, enabling us to make more informed decisions across various fields like finance, economics, or marketing.
Cyclical analyses help to predict potential outcomes and optimize strategies based on recurring events. It allows us to make better-informed decisions and build more robust models. In short, cyclical analysis provides valuable insights that can help inform decision-making.
It is an essential tool for understanding datasets and uncovering the underlying relationships at play. It helps us to identify hidden patterns and capture the complete picture. By combining trend and cyclical analyses, we can make more informed decisions and develop better strategies for various fields.
This holistic approach enables us to obtain a comprehensive understanding of data, allowing us to extract important insights that may have been missed otherwise.
Understanding Cyclical Analysis
To effectively apply cyclical analysis, it is crucial to differentiate between trend and cyclical behavior in datasets. Trends represent long-term movements in data, reflecting its general direction over an extended period. In contrast, cyclical behavior involves shorter-term repetitive patterns that occur within the trend, often influenced by seasonal or periodic factors.
Human Tendency to Focus on Trends and the Pitfalls of Ignoring Cyclicality
As humans, we have a natural inclination to focus on trends due to their apparent significance and long-term impact on data. While trends provide valuable insights, disregarding cyclical behavior can lead to misconceptions and incomplete assessments.
Ignoring cyclical patterns may result in overlooking crucial short-term and long-term variations, limiting predictions’ accuracy and decision-making processes.
Emphasizing Mathematics Over Mysticism: No Magic, Only Observational Analysis
It is essential to dispel any notion of mysticism surrounding cyclical analysis. Cyclical analysis is firmly grounded in mathematical principles and observational analysis.
It involves rigorous examination and mathematical modeling of data to identify recurring patterns. No element of magic or fortune-telling is involved; rather, it is a systematic and data-driven approach to understanding cyclicality.
Addressing Misconceptions: Differentiating Between Prediction and Relationship Spotting
One common misconception about cyclical analysis is that it is a form of predicting the future with certainty. However, it is crucial to clarify that cyclical analysis does not predict specific future events. Instead, it focuses on spotting mathematical relationships and patterns that have occurred and are likely to repeat in the future under similar conditions.
By identifying such relationships, cyclical analysis provides a framework for understanding the potential behavior of a system within a certain timeframe. It equips analysts with the knowledge to make more informed assumptions and predictions but does not offer absolute certainty. Emphasizing this distinction is essential to avoid misunderstandings and the promotion of unrealistic expectations associated with cyclical analysis.
Who knows, maybe one day, a fancy mathematical equation will be able to tell us the name of the tennis player who will win Wimbledon or that a specific company will have a horrible first quarter next year. Until then, cyclical analysis remains our best tool to get to know a dataset without the exact knowledge of advanced mathematical equations.
The Mechanism Behind Cyclical Analysis
Cyclical analysis allows us to delve into the intricate web of nature’s unexplored relationships. Many natural phenomena and human activities exhibit cyclical patterns that are not immediately evident. By applying cyclical analysis to various datasets, we can uncover these hidden connections, gaining a deeper understanding of how different factors interact over time.
Multi-dimensional Equations as Tools for Relationship Identification
Central to cyclical analysis are multi-dimensional equations, which serve as powerful tools for identifying relationships within datasets. These equations can capture complex interactions between multiple variables and reveal cyclical patterns that might not be apparent through simpler methods. Using such equations enhances our ability to model and interpret data, enabling us to grasp the underlying dynamics contributing to cyclicality.
The Potential of Advanced Mathematical Models in Explaining Complex Phenomena
Cyclical analysis is indispensable when dealing with complex phenomena that resist simple linear interpretations. As datasets grow more intricate, advanced mathematical models play an increasingly vital role in deciphering the underlying relationships. These models have the potential to explain intricate patterns and fluctuations in data, allowing us to gain deeper insights into the mechanisms driving cyclical behavior.
While the quest for complete explanations may continue, cyclical analysis is our best current method to spot mathematical relationships within data. These relationships offer valuable insights that can be harnessed for prediction and decision-making, even if we do not comprehensively understand all contributing factors. As technology and analytical methodologies progress, the potential for explaining and predicting complex phenomena through cyclical analysis becomes even more promising.
The Role of Cyclical Analysis
Cyclical analysis is pivotal in identifying and understanding mathematical relationships within datasets. By meticulously observing and analyzing data over time, we can detect patterns that repeat at regular intervals. These observed cyclical patterns indicate the presence of underlying mathematical relationships that govern the behavior of the system being studied. Through this process, cyclical analysis provides valuable insights into the data’s fundamental dynamics.
Applications in Various Fields
The versatility of cyclical analysis allows its application across diverse fields. In finance, it can be utilized to recognize market cycles, understand economic fluctuations, and optimize investment strategies: a great helper for any trader. In economics, cyclical analysis aids in identifying business cycles, consumer behavior patterns, and the impact of economic policies.
Even in sports, cyclical analysis can uncover performance patterns, assess player form, and anticipate favorable conditions for specific events.
Spotting Cycles and Understanding Repeating Patterns
One of the key roles of cyclical analysis is to spot cycles and comprehend repeating patterns in data. Seasonal changes, natural phenomena, or other recurring events may influence these cycles.
By identifying and comprehending these patterns, analysts can make more informed predictions, anticipate potential outcomes, and devise strategies that align with the cyclical nature of the system. Understanding cycles empowers decision-makers to capitalize on advantageous phases and mitigate risks during challenging periods.
In essence, the role of cyclical analysis extends beyond mere data interpretation; it empowers professionals across various fields to leverage the observed patterns for better decision-making and planning. As cyclical analysis continues to evolve with advances in data science and mathematical modeling, its applications are likely to expand, becoming an increasingly valuable tool for understanding and harnessing the cyclical nature of the world around us.
Determining the Feasibility of Cyclical Analysis
The feasibility of cyclical analysis heavily depends on the strength of trend components in the dataset. When the trend component dominates the data and masks the cyclical patterns, conducting effective cyclical analysis becomes challenging.
In such cases, the cyclical signals may be obscured, making extracting meaningful insights from the data difficult. Understanding the interplay between trend and cyclical behavior is crucial for accurate analysis and interpretation.
Conditions for Successful Cyclical Analysis
Several conditions contribute to the success of cyclical analysis (click on each element below for additional details):
Cyclical patterns often occur over relatively short timeframes. Having a dataset with adequate resolution, such as frequent data points, enhances the ability to accurately spot and analyze these cycles.
For successful cyclical analysis, the cycles should exhibit a consistent pattern with clear periodicity. Regular cycles that repeat predictably enable more robust analysis and reliable predictions.
If the dataset contains a strong trend component, pre-processing techniques may remove or dampen its influence, enabling a clearer view of the cyclical patterns.
Understanding the domain being studied is vital. Knowledge of relevant factors that could influence cyclical behavior helps interpret results accurately and avoid false conclusions.
Practical Examples of Effective Cyclical Analysis
Let us mention a few practical applications of cyclical analysis (click on each element below for additional details):
Retail businesses often experience cyclical fluctuations in sales due to seasonal factors. Cyclical analysis helps identify peak periods, such as holiday seasons, allowing businesses to optimize inventory and marketing strategies accordingly.
Economists study cyclical variations in economic indicators like GDP, unemployment rates, and consumer spending. Identifying these business cycles assists in formulating economic policies and predicting potential economic downturns or upswings.
Meteorologists use cyclical analysis to study weather patterns like El Niño and La Niña, which occur cyclically and impact global weather conditions. Understanding these patterns aids in long-term climate predictions and disaster preparedness.
By assessing the strength of trend components and applying appropriate analytical techniques, cyclical analysis can be a powerful tool for revealing hidden patterns and relationships in data. When conducted under suitable conditions with domain knowledge and precise data resolution, cyclical analysis enhances our ability to make informed decisions and predictions, ultimately improving our understanding of cyclical phenomena in various domains.
Advantages and Limitations of Cyclical Analysis
Like any analytical approach, cyclical analysis has both advantages and limitations. Understanding these aspects is crucial to harnessing its potential effectively. Analysts can make well-informed decisions and extract valuable insights from datasets by acknowledging the benefits of this strategy and addressing its limitations.
Benefits and Limitations of Recognizing Cyclical Behavior in Datasets
By identifying cyclical patterns, analysts can make more accurate short-to-medium-term predictions, anticipating future occurrences based on historical data. This can be particularly beneficial in fields like finance, where recognizing market cycles can lead to more profitable investment decisions.
Recognizing cyclical trends enables businesses and organizations to allocate resources more efficiently. For instance, adjusting staffing levels or production schedules based on cyclical fluctuations in demand can reduce costs and enhance productivity.
In areas heavily influenced by seasonal changes, such as agriculture and tourism, cyclical analysis assists in planning and preparation for seasonal variations, ensuring businesses and industries can adapt to changing conditions.
Understanding cyclical behavior allows decision-makers to align their strategies with the cyclical nature of the data, resulting in more informed and targeted actions that can lead to better outcomes.
Cyclical patterns may be obscured or indistinguishable in datasets dominated by strong trend components. This can lead to incomplete or inaccurate analysis if cyclical behavior remains undetected.
Making accurate predictions can be challenging if the cyclical signals are weak or irregular. Decision-makers must exercise caution when relying solely on cyclical analysis in such cases, as it may result in unreliable predictions.
Applying cyclical analysis to datasets with insufficient resolution or a limited historical range may lead to overfitting, where models perform well on historical data but fail to generalize to new data.
In datasets with multiple interacting cyclical patterns, disentangling the various cycles and understanding their contributions can be complex and require sophisticated analytical approaches.
Cycle Quest – Your Partner in Cyclical Analysis
Cycle Quest offers personalized cyclical analysis services tailored to businesses, investors looking to improve their trading, and curious learners’ needs. Their team of knowledgeable advisors specializes in various industries, ensuring expertise in sourcing and analyzing the best datasets.
Here is the simple 3-step process you need to know before contacting Cycle Quest:
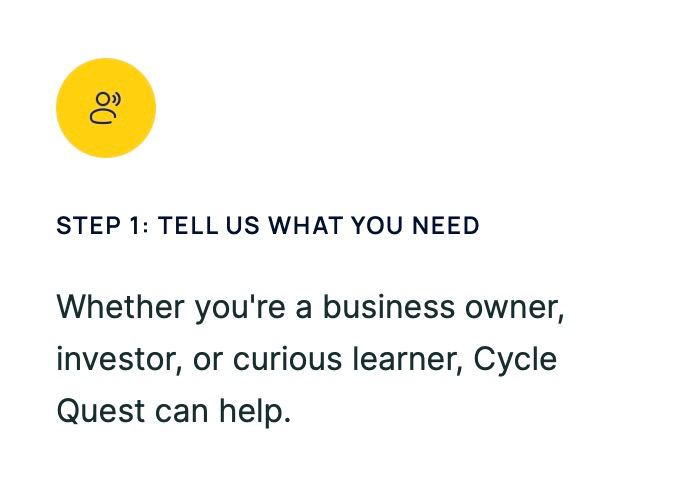
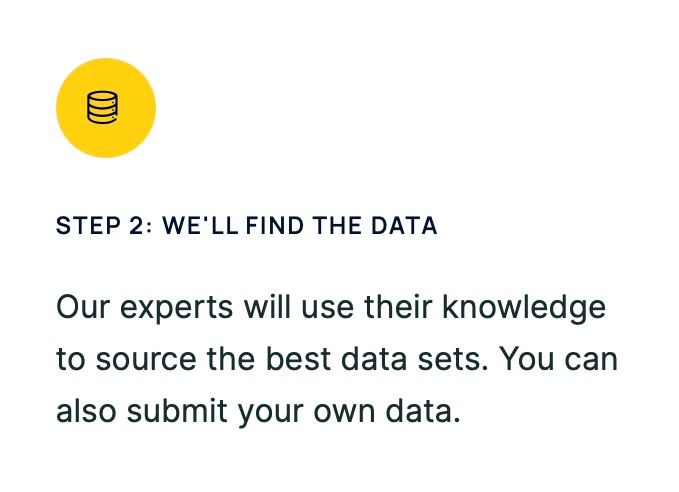
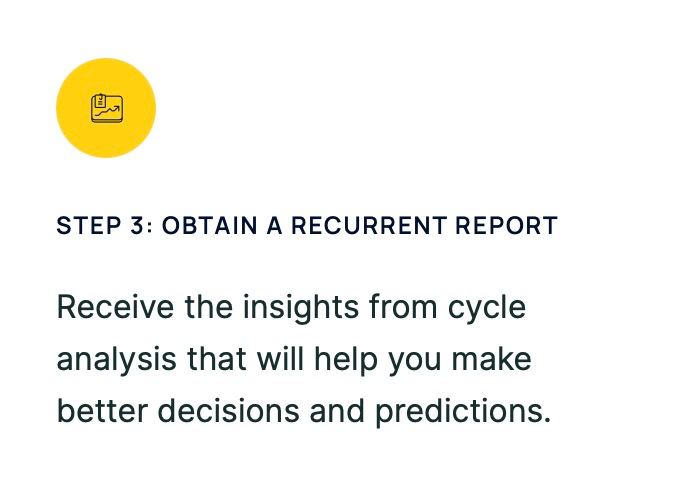
Contact us to explore successful examples of cyclical analysis applications across diverse areas like business, investments, sports, and science.
Let Cycle Quest be your guide in unraveling the hidden cyclical patterns within your data, enabling you to gain a competitive edge and optimize your strategies.